AI/ML integration is soon to become an existential issue
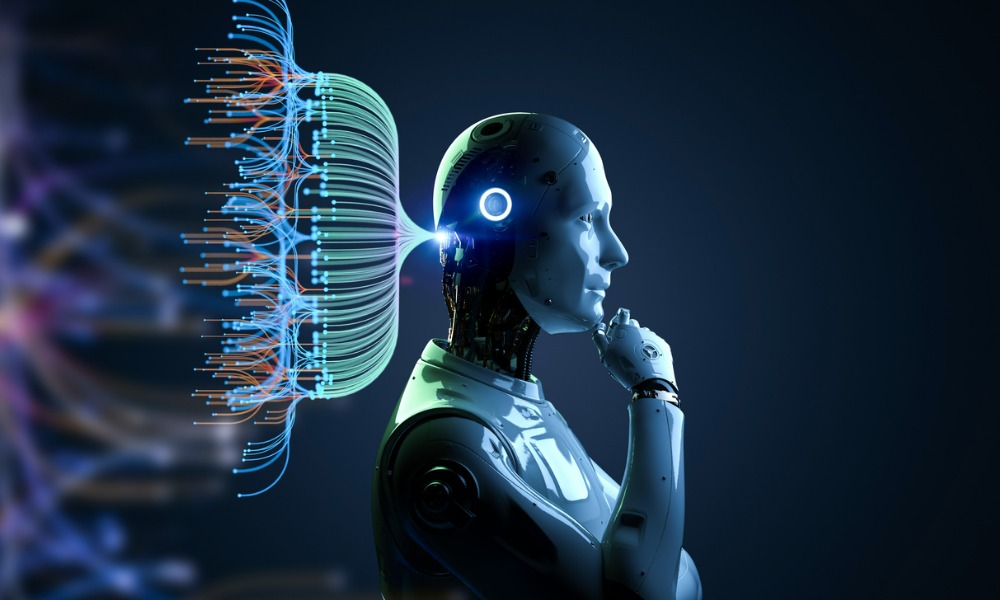
Machine learning algorithms are increasingly being used in the banking industry to forecast economic growth and make informed decisions, yet the practice is an abstraction at best for many.
Brian Sathianathan, co-founder of Iterate.ai, helps demystify the practice. He detailed the many ways in which ML is being used in the banking sector.
Consumer banking is one key area for ML
“Consumer banking is a key area where ML is applied,” Sathianathan said. “By analyzing consumer data, such as credit card transactions and spending patterns, banks can predict consumer behavior and tailor their products and services accordingly.”
One aspect of ML is to predict consumer behavior: “For example, a bank can use machine learning to identify customers who are likely to default on their loans and take appropriate action to prevent it,” he noted.
Another focal point for ML: Operations
“Operations is another area where machine learning is used in banking,” the co-founder said.
“Machine learning algorithms can be used to automate routine tasks, such as processing loan applications and detecting fraud. This not only improves the efficiency of the bank but also reduces the chances of errors.”
Forecasting economic growth
“Machine learning can also be used to forecast economic growth,” Sathianathan added. “By analyzing data on various economic indicators, such as GDP, inflation, and unemployment rates, machine learning algorithms can predict the direction of the economy. This information can be used by banks to make informed decisions on investment and lending.”
In those three areas – consumer banking, operations and economic growth forecasting – ML is now playing a “crucial role” in the banking sector, he noted. “With its ability to accurately predict economic indicators, machine learning algorithms are helping banks make informed decisions and drive economic growth,” he added.
Integration soon to be an existential issue
A survey by Cloudera showed that artificial intelligence and machine learning capabilities are both poised to “revolutionize” business processes due to an explosion of data and exponential increase in computing power. “But many of the organizations working with AI/ML models today do not have a data strategy in place to support it,” officials wrote. “The effective integration of AI/ML models will soon be an existential issue.”
According to the study, 92% of organizations are working with AI/ML models but 61% don’t have a data strategy in place to support it. “Thanks to an explosion of data, exponential increases in computing power and storage capacity, and better algorithms, artificial intelligence (AI) and machine learning (ML) capabilities are poised to revolutionize business processes,” the study’s authors wrote.
“There is a lot of long-term momentum in the AI market, said Nitish Mittal, vice president in the digital transformation practice at global research firm Everest Group. He noted AI/ML adoption is expected to accelerate through 2024.
Many organizations see minimal impact
Wayne Butterfield, director and leader of the European AI automation practice at business consultancy and research firm ISG, said a limited number of organizations are seeing significant business impact from their AI/ML investments so far. “If we break down AI into its various components, nearly every enterprise is using one of them,” he said. “But none of it is transformative. It’s very much happening around the edges. Very few organizations are AI-first. Most organizations are not set up to achieve that.”
Most organizations are in the early stages of their application of AI/ML models, according to the research. Results are more likely to accrue incrementally over the next few years — a gradual transformation that will ultimately become part of the fabric of leading companies, analysts found.
“It will be more like a slow birth than a sudden overnight shift,” Butterfield said. “This low-speed transformation will require significant determination and coordination. “Transformation using AI requires a collection of people, departments, technology types, and more all coming together under a single umbrella,” he added. “It is going to be difficult.”