Altos Research verifies ‘Likely to Move’ 19.5 percent prediction accuracy
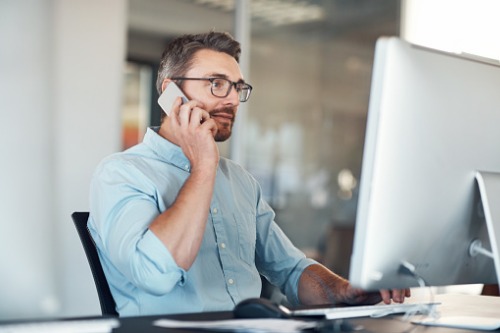
You’ve completed your morning routine, you’ve time blocked the next eight hours, you have the opportunity to reach out to 20 clients before you move on to the next task.
With more than 2,000 people in your database, how do you know the right people to call?
Automation helps, using programs that constantly watch mortgage rates for refinance opportunities. Another option, Revaluate, came onto the scene in 2017 using predictive analytics that look “the Data Ds’ that cause people to move” in the next three to six months. In a recent study, Altos Research found Revaluate’s “likely to move” prediction success rate to be 19.5 percent.
“This is by far the best lead success rate we’ve seen in the real estate industry. We also expect the rate to improve over time, given the machine learning Revaluate’s algorithms employ,” said Mike Simonsen, CEO of Altos Research, the independent third party that was commissioned to evaluate Revaluate’s prediction accuracy.
While 19.5 percent might not sound particularly high, think about it this way: of those 20 clients that a mortgage originator has time to call on any given day, one is a bona fide hit. Everyone’s heard of the big life events that usually spark a move, also known as “The Four Ds”: Diamonds, Diapers, Divorce, and Death. But Revaluate also adds some new Ds to the mix, including: the Daily Grind, which involves job-related moves; Diplomas, which could involve a child moving out and needing help purchasing a property of their own; and Downsizing, when retirees no longer need or want a large space and look to move to a smaller home.
Simonsen said that that success rate would be higher if there was a reliable source, such as the MLS, through which they could have compared the home buyers and renters identified by Revaluate as “likely to move.” But 19.5 percent is actually a “significant” improvement over the randomness of everything else in the world, Simonsen said, especially when it comes to human behavior. And, he adds, that prediction success rate is only going to get more accurate as time goes on.
“A couple things can happen when you’re building the predictive models. One is, you have a longer period of history to build a model from,” he said. “The longer of history you have, the more accurate the models get. The interesting trade-off is that you need to have a big set of data that you didn’t build the model from, a new set of data that you can test the model with to know if it’s any good. So the better your test is, the worse your model is, because you have this finite amount of data, or the better your model is, the worse your test is because you have to take some of it outside your set and save it for testing later.”
Altos Research has been around for 13 years, and has been gathering data since its inception. There are hundreds of different MLSs across America, some of which are very sophisticated, but they are very localized and weren’t created in order to facilitate a completely amalgamation of data and analysis.
“With real estate there’s actually not that much data, and then on top of it, nobody even kept any data until 13 years ago, when we started. It’s crazy,” Simonsen said. “We now have this super rich data set, so now we can go back and test for years to that a company like Revaluate can know if they’re actually predicting anything worthwhile.”
Revaluate was founded with a mission to help real estate and mortgage professionals and those in adjacent industries improve their sales effectiveness and productivity by more accurately predicting who will move, so that they can better target their sales and marketing efforts to those people. The company focuses on using the prospects that already exist within someone’s database, and applies its proprietary algorithms and artificial intelligence to analyze the likelihood that someone will move—and need to find a new home or get a new mortgage.